```python #!pip3 install tensorflow import tensorflow as tf import numpy as np import matplotlib.pyplot as plt ``` ```python # data for training the ann mode # option 1: celsius = np.array([-40, -10, 0, 8, 15, 22, 38], dtype=float) fahrenheit = np.array([-40, 14, 32, 46, 59, 72, 100], dtype=float) # option 2: (X°C x 9/5) + 32 = 41 °F points = 100 np.random.seed(99) dataIn = np.linspace (-40,60, points) target = dataIn*9/5 + 32 +4*np.random.randn(points) plt.plot(celsius, fahrenheit, 'or', label='data-set 1') plt.plot(dataIn, target, '.b', alpha=0.3, label='data-set 2') plt.legend() plt.grid() plt.show() ``` 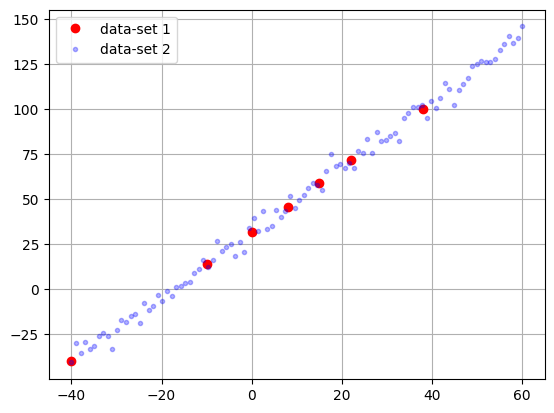 ```python from tensorflow.keras.models import Sequential # ANN type from tensorflow.keras.layers import Dense, Input # All nodes connected # NN definition hn=2 model = Sequential() model.add(Input(shape=(1,), name='input')) model.add(Dense(hn, activation='linear', name='hidden')) model.add(Dense(1, activation='linear', name='output')) model.summary() ```
Model: "sequential_1"
┏━━━━━━━━━━━━━━━━━━━━━━━━━━━━━━━━━┳━━━━━━━━━━━━━━━━━━━━━━━━┳━━━━━━━━━━━━━━━┓ ┃ Layer (type) ┃ Output Shape ┃ Param # ┃ ┡━━━━━━━━━━━━━━━━━━━━━━━━━━━━━━━━━╇━━━━━━━━━━━━━━━━━━━━━━━━╇━━━━━━━━━━━━━━━┩ │ hidden (Dense) │ (None, 2) │ 4 │ ├─────────────────────────────────┼────────────────────────┼───────────────┤ │ output (Dense) │ (None, 1) │ 3 │ └─────────────────────────────────┴────────────────────────┴───────────────┘
Total params: 7 (28.00 B)
Trainable params: 7 (28.00 B)
Non-trainable params: 0 (0.00 B)```python ### veri important note implement a python code # to show the ANN model connection using ascii ``` ```python from tensorflow.keras.optimizers import Adam #hyper parameters epoch = 500 lr = 0.01 hn = 2 # hidden nodes tf.random.set_seed(42) # For TensorFlow model.compile(optimizer=Adam(lr), loss='mean_squared_error') print("Starting training ...") historial = model.fit(dataIn, target, epochs=epoch, verbose=False,) print("Model trainned!") ``` Starting training ... Model trainned! ```python predict = model.predict(dataIn) plt.plot(dataIn, predict, ':r', label='estimated') plt.plot(dataIn,target, '.b', label='real', alpha=0.4) plt.legend() plt.grid() plt.show() ``` [1m4/4[0m [32m━━━━━━━━━━━━━━━━━━━━[0m[37m[0m [1m0s[0m 4ms/step 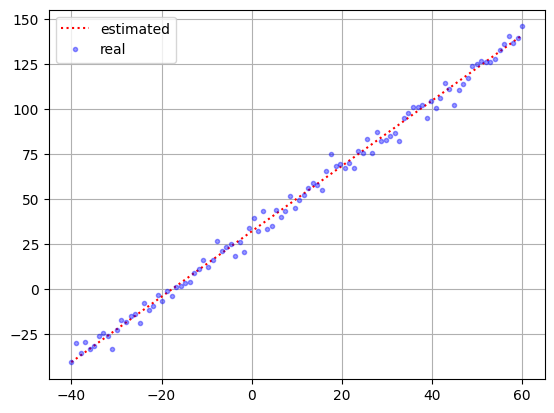 ```python # Get weights for layer in model.layers: weights = layer.get_weights() print(f"Layer: {layer.name}") print(f" Weights (Kernel): {weights[0].shape} \n{weights[0]}") print(f" Biases: {weights[1].shape} \n{weights[1]}") ``` Layer: hidden Weights (Kernel): (1, 2) [[-0.27738443 0.7908125 ]] Biases: (2,) [-8.219968 6.714554] Layer: output Weights (Kernel): (2, 1) [[-1.9934888] [ 1.5958738]] Biases: (1,) [5.1361823] # Testing the model ```python inTest = np.array([100]) model.predict(inTest) ``` [1m1/1[0m [32m━━━━━━━━━━━━━━━━━━━━[0m[37m[0m [1m0s[0m 87ms/step array([[213.73816]], dtype=float32) ```python # Do the Maths: inTest = np.array(inTest) whi = np.array([[-0.27738443, 0.7908125 ]]) bh = np.array([-8.219968, 6.714554]) Oh = np.dot(inTest,whi)+bh who = np.array([[-1.9934888],[ 1.5958738]]) bo = np.array([5.1361823]) Oo = np.dot(Oh,who)+bo Oo ``` array([213.73814765]) ```python def generate_ascii_ann(model): ascii_diagram = "\nArtificial Neural Network Architecture:\n" for i, layer in enumerate(model.layers): weights = layer.get_weights() # Determine layer type and number of neurons if isinstance(layer, Dense): input_dim = weights[0].shape[0] # Number of inputs output_dim = weights[0].shape[1] # Number of neurons ascii_diagram += f"\nLayer {i+1}: {layer.name} ({layer.__class__.__name__})\n" ascii_diagram += f" Inputs: {input_dim}, Neurons: {output_dim}\n" ascii_diagram += f" Weights Shape: {weights[0].shape}\n" if len(weights) > 1: # If bias exists ascii_diagram += f" Biases Shape: {weights[1].shape}\n" # ASCII representation of neurons ascii_diagram += " " + " o " * output_dim + " <- Output Neurons\n" ascii_diagram += " | " * output_dim + "\n" ascii_diagram += " " + " | " * input_dim + " <- Inputs\n" return ascii_diagram # Generate and print the ASCII diagram ascii_ann = generate_ascii_ann(model) print(ascii_ann) ``` Artificial Neural Network Architecture: Layer 1: hidden (Dense) Inputs: 1, Neurons: 2 Weights Shape: (1, 2) Biases Shape: (2,) o o <- Output Neurons | | | <- Inputs Layer 2: output (Dense) Inputs: 2, Neurons: 1 Weights Shape: (2, 1) Biases Shape: (1,) o <- Output Neurons | | | <- Inputs ```mermaid graph LR I1((I_1)) --> H1((H_1)) & H2((H_1)) H1 & H2 --> O1((O_1)) & O2((O_2)) ```